Generative AI Model Inputs: Implications for AI Regulatory Compliance
With the growing interest in AI, financial firms looking to jump on board must become more familiar with AI regulatory compliance. The governance of generative AI must include an examination of the inputs and outputs of large language models (LLM), as well as the algorithms and logic inside the models themselves.
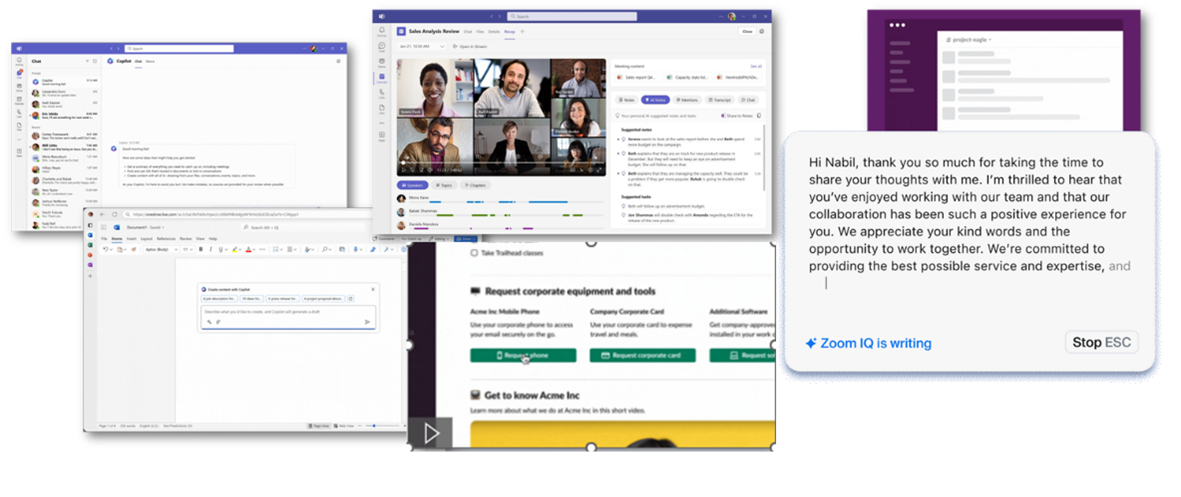
Model inputs are an underappreciated area of analysis in the use of generative AI for risk and compliance. In fact, the effectiveness of generative AI is highly correlated with the quality of the data that serves as input into models.
These model inputs are often drawn from previous interactions with customers, which increasingly include communications tools such as:
- Social media applications
- Conferencing technologies like Zoom, as well as SMS and text messaging
- Internal collaboration tools (e.g., Slack and Microsoft Teams) that may include voice, video, and use of emojis
Complicating this is the fact that many of these tools are embedding OpenAI (or comparable technologies) into their platforms, which require analysis and due diligence. In short, content sources that can be used to feed models are not homogeneous, and each feature, function, or unique metadata can impact the effectiveness of a model.
Key considerations for firms to evaluate for AI regulatory compliance include:
- How each content source exposes non-message features to enable capture and consumption for the potential use of generative AI applications
- How existing control applications (e.g., recordkeeping, supervisory, and surveillance) can index, store, and normalize unique collaborative features for use within generative AI models
- What data protections each vendor provides to ensure that data privacy and intellectual property obligations have been adhered to
- Whether those vendors use customer information in training their models, as represented in opt-in/-out features and consent policies
- How applications provide notification of new features or capabilities that may impact the ability to consume content from those sources and model agility
- How commercialization models may evolve as content vendors prioritize their own proprietary models
Governing the output of generative AI applications
Governance decisions about what model outputs should be captured, retained, and surveilled are complex. You must account for differences in regulatory jurisdiction, including the status of an individual or type of firm that is providing the application and whether the application is built with a publicly accessible or closed/proprietary model.
As a general AI governance framework for model outputs, firms should consider three primary variables:
- Who has access to the model output
- What use case the model is serving
- How that output aligns with existing regulatory obligations based on its value and potential risk to the firm
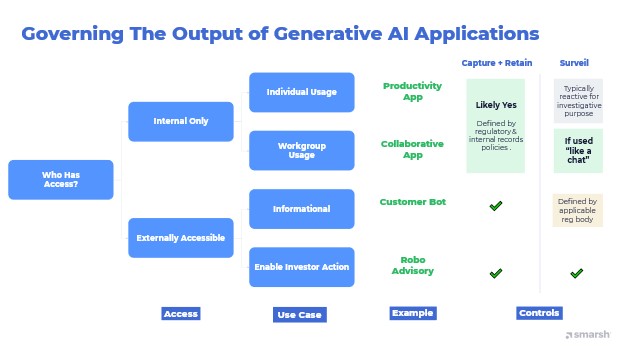
Internal-only access
The upper branch represents tools or applications where the output is being used exclusively for internal use and where there is no access to the output external to the firm. Here, one should consider the use case and whether the application is used solely by an individual or multiple employees or workgroups.
Individual usage: An example of this use case is an employee interacting with the application itself, such as for internal research for research reports or writing first drafts of internal documents.
- Control considerations: Capture and retention decisions are driven by firm policy and the retention obligations mandated by financial sub-verticals or other industries. Supervision and surveillance will likely center on investigating a specific issue, whether related to regulatory or internal policy issues. Firms should evaluate their ability to re-create an event (including the prompt and associated output) if choosing not to capture this content proactively.
Workgroup usage: This is a use case of generative AI applications used by a group of employees where the output may be shared and potentially lead to a business-related decision. Scenarios include the use of AI embedded into Microsoft Teams, where that functionality is used to help reach a decision that potentially could be shared as part of a product offering or some other capability.
- Control considerations: This use case highlights the complexity of interpreting records retention obligations for collaborative features where explicit regulatory guidance is lacking. While differences exist across regulatory bodies, and specific employee roles do need to be considered (e.g., FINRA-regulated broker-dealers), this question can be reduced to whether the output adds potential value or risk to the business. Collaboration adds risk because of the potential for collusive or other prohibited internal insider activity, and firms should pattern surveillance practices after existing policies that govern collaborative applications.
Externally accessible access
The external branch addresses generative AI-enabled applications that are accessible outside of the firm in interacting with prospects/clients or the market. Use cases here are divided between applications used for information purposes only versus those that enable action from a prospect or client.
Informational use case: This use case addresses the delivery of information. This can include helping to automate the search and retrieval of basic investing information, such as providing answers via a customer bot.
- Control considerations: Communications with the public carry specific obligations to capture and retain, which vary by specific sub-vertical market. In most cases, firms would define these as business records and, therefore, require the capability to capture and retain that information. Supervision and surveillance of this capability would first depend on the firm’s or individual’s specific regulatory mandates, such as the need to provide written supervisory procedures (WSPs) for broker-dealers requiring proactive oversight.
Enabling action use case: The final scenario is the simplest to define as it intersects with a variety of existing regulations that provide investor protection. It requires the highest level of transparency by the firm as it enables investor action. This use case includes providing an advisory service, which most major firms have provided with robo-advisory services for multiple years.
- Control considerations: Enabling action via services directly impacts the capture and retention obligations faced by most firms. It touches the firm's responsibility to monitor the output of automated tools by providing a human interface as a co-pilot to ensure that information delivered to an external audience is evaluated before it is delivered.
Key takeaways
Generative AI represents a disruptive force in financial services and a potential source of information risk that requires proactive governance. As a start with AI regulatory compliance, firms need to:
Understand their models
Firms must understand their models, including the inputs used, where information comes from, and what information protections are built into the inputs being generated.
Best practices for model governance and risk management are both emerging arenas. Compliance teams need to work with their internal data science teams to understand the inner workings of the application to the level required to defend its use with regulators. This includes how it is being managed to address bias and the steps the firm is taking to ensure that information is properly managed.
Know how outputs touch regulation
Firms need to map applications touched by generative AI against policies, both in terms of internal communications against internal policies and externally for applications that deliver information or enable decisions by investors’ interactions. As suggested by the model, some of these decisions are not straightforward. A risk-based approach can help guide policy decisions based on the impact and consequences of information that may be delivered inappropriately.
Maintain due diligence of external applications
Firms need to prioritize internal development and the applications you already have in support of your organization. Every leading communication and collaborative application will likely be embedding generative AI into its products in the near future. Knowing how those vendors manage information and prioritize data privacy and ownership rights can only become more critical in the near term.
Share this post!
Smarsh Blog
Our internal subject matter experts and our network of external industry experts are featured with insights into the technology and industry trends that affect your electronic communications compliance initiatives. Sign up to benefit from their deep understanding, tips and best practices regarding how your company can manage compliance risk while unlocking the business value of your communications data.
Ready to enable compliant productivity?
Join the 6,500+ customers using Smarsh to drive their business forward.
Subscribe to the Smarsh Blog Digest
Subscribe to receive a monthly digest of articles exploring regulatory updates, news, trends and best practices in electronic communications capture and archiving.
Smarsh handles information you submit to Smarsh in accordance with its Privacy Policy. By clicking "submit", you consent to Smarsh processing your information and storing it in accordance with the Privacy Policy and agree to receive communications from Smarsh and its third-party partners regarding products and services that may be of interest to you. You may withdraw your consent at any time by emailing privacy@smarsh.com.
FOLLOW US